1. INTRODUCTION
Computational science is a new interdisciplinary field commonly used to explore problems that are difficult to approach using conventional theories or experimental simulations (Cho 2016b). As computers have evolved, computational science has become a more economical method of solving such problems, and its popularity has increased (Cho 2016b). Supercomputers and massive amounts of data help overcome human limitations (Lin & Yen 2009;Cho 2011;Cho et al. 2015;Cho 2016a) and computational science helps researchers and experts create theoretical models of complicated, expensive, dangerous, large-scale, and hyperfine phenomena (Cho 2016b). They help us to model the origin of the universe using simulations, and provide the only way for us to observe its evolution (Cho 2016b).
While the Standard Model of particle physics was completed with discovery of the Higgs boson (ATLAS collaboration 2012;CMS collaboration 2012), it is thought to be imperfect as a theory because gravitational force was excluded from the equation. Newton’s equations of motion can be applied to the natural world, but yield results that deviate from common sense in the range of the speed of light, including a mass increase and a length decrease. Likewise, the Standard Model has proven to be accurate for describing phenomena at the current energy scale, but is likely to be unsuitable at higher energy scales.
It is known that the universe today consists of 4 % Standard Model particles, 26 % dark matter, and 70 % dark energy (Cho 2016a). Specifically, dark matter research is increasingly reliant on computational science due to changes in the research environment and constant upgrades of data, facilities, equipment, and computing capacity.
Here, we introduce a methodology for dark matter research based on data, computational science, and deep learning. We suggest a dark matter research platform and discuss its role in modern science.
2. DARK MATTER DETECTION
To date, the known conditions required for dark matter are that it emits no type of light, escapes and does not collide with any matter, and is a cold model that was close to zero velocity in the early universe (Cho 2016b). Methods of detecting dark matter can be classified into direct detection, indirect detection, and accelerator detection. Fig. 1 shows the three methods of detecting dark matter and the interactions between the Standard Model and dark matter.
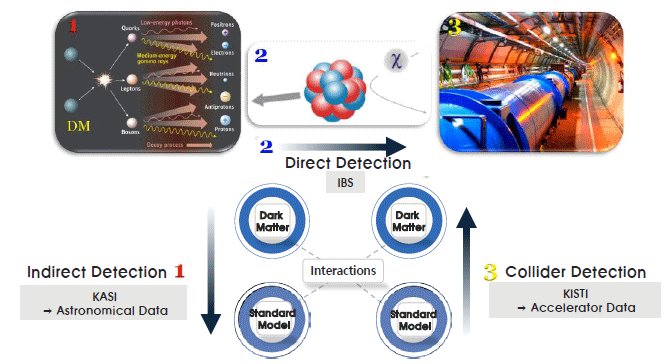
The first method is indirect detection. In cosmology, dark matter interacts and produces Standard Model particles. The Korea Astronomy and Space Science Institute (KASI) provides large-scale cosmology data for the indirect detection of dark matter.
The second method is direct detection. Since dark matter has mass, it interacts with detectors. The Institute for Basic Science (IBS) is currently performing underground direct detection and Axion experiments.
The third method is accelerator detection. Using the Standard Model particle collider with an accelerator, we can generate possible dark matter. The Korea Institute of Science and Technology Information (KISTI) provides accelerator data for researchers in Korea who use it to search for dark matter.
Combining research on dark matter using computational science-based particle physics and that using cosmology increases the synergy effect between them. Regarding the infrastructure of each organization, the KASI uses the mass data center and supercomputer at the KISTI for proposed model research in cosmology. The KISTI also stores data observed at the KASI to compare with experimental data. To promote efficient national research, the development of calculation science, and the synergy effect of research, a dark matter research platform is established to allow resource and information sharing between researchers.
Fig. 2 shows the locations of astronomical and accelerator data retrieval. For astronomical data, Sloan digital sky survey (SDSS) dark energy spectroscopic instrument (DESI) data are produced in the USA and large synoptic survey telescope (LSST) are produced in Chile. For accelerator data, compact muon solenoid (CMS) data is produced at European Organization for Nuclear Research (CERN) in Europe, Belle data is produced at High Energy Accelerator Research Organization (KEK) in Japan, and Deep Underground Neutrino Experiment (DUNE) data is produced at Fermilab in USA. Table 1 shows the limitations of data sharing for astronomical data, accelerator data, and simulation data.
3. METHODOLOGY
In this section, we consider what we need for dark matter research. This is explained from the point of view of data, computational science, and deep learning. Regarding data, usage is moving from a theory-driven approach to a data-driven approach. However, the value of data over time drives automated low latency actions in response to events of interest. Big data employ a large historical dataset to make split second decisions on real time data, as shown in Fig. 3.
Data analysis has also changed from a theory-driven approach to a data-driven approach. The theory-driven approach, by which the Higgs boson was discovered (CMS collaboration 2012;ATLAS collaboration 2012), starts with the system and works towards the data. The data-driven approach starts with the data and works towards the system. Tables 2 and 3 show the planned future surveys of accelerator data (Song 2016). The size of LSST data in 2023–2030 will be 500 PB. The size of accelerator data will also increase enormously. Large hadron collider (CMS) data will be 100 PB/yr, which is 5–10 times its current size. Therefore, we need to prepare the system for this increased data size.
Computational science represents the convergence of theory, experiments, and simulations (Cho 2016b). Here, we describe the dark matter research requirements from each point of view. Regarding theory, requirements include rapid updates and sharing of information, a specialist key point summary database, regular meetings for network feedback between experimentalists and theorists, rapid and easy comparisons of theoretical models, development of new numerical packages and computing power, and cross checks with astrophysics regarding theoretical models such as the N-body simulation. Regarding experiments, requirements include manpower for dark matter research using hardware and software, social networking at local and international institutions related to indirect, direct, and accelerator detection experiments, regular workshops for communication between the experimental and theoretical community, and big data computing resources for high-performance computing (HPC) and storage of experimental data. Regarding simulations, a large number of simulations with a theoretical background are required for dark matter detection, in which speed or memory issues are considered (Cho 2016b). Therefore, it is necessary to develop simulation tool kits (Cho 2016b).
This research cannot be performed by an individual or single research organization. The reason computational science is required in dark matter research is that the number of signal cases is small owing to the small cross-section (Cho et al. 2015). The Standard Model was established due to the discovery of the Higgs boson, which has the smallest scattering cross-section among standard models. However, the cross-section of dark matter is less than one thousandth that of the Higgs boson (Cho 2016b). Therefore, at least 1,000 times more experimental and simulation data are required than for the Higgs boson (Cho et al. 2015). In addition, the mass range and coupling constant range are wide (Baer et al. 2015). For dark matter simulation, a dark matter research management system is required. By calculating the database and parameters according to the model, the experimental results of this system can restrain theoretical parameters and enhance precision of predicted values. With an automated tool, researchers can easily obtain quicker and more accurate results. The main goals are to develop a simulation toolkit involving a phenomenological analysis technique for large-scale simulation and testing of candidate dark matter models, and to conduct applied studies using the developed toolkit that are available to the wider research community. Software needs to be developed and applied to meet the demands of the evolving physics. This can be done by conducting a combined reconstruction of relevant components to simulate candidate dark matter models.
The third aspect of dark matter research is deep learning. Higgs boson detection has been applied to deep learning in High Energy Physics (Baldi et al. 2014). The DUNE experiment uses image processing. Fig. 4 shows the process of deep learning. From the theoretical model, we generate Monte Carlo events using the KISTI supercomputers and compare the results with experimental data. The results are used as input data for the deep learning process.
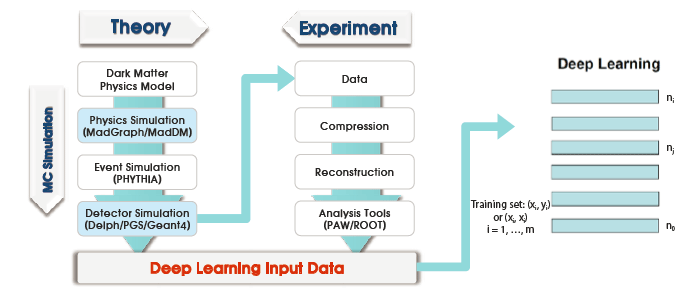
As well as the requirements for dark matter research discussed above, astronomical data and accelerator data must be combined to make the processing and analysis more efficient. Using deep learning, we can process and analyze convergence data using a big data platform and research and development (R&D) for Intelligence Information to release output data. Fig. 5 shows the suggested dark matter research platform. The KISTI processes accelerator data, and the KASI processes astronomical data, using deep learning and put these data on the dark matter research big data platform. Simulation data is produced through the KISTI supercomputer using theory. We compare simulation data with astronomical data using deep learning. The dark matter research platform for big data consists of a deep learning and named data networking (NDN) system. Dark matter researchers can then use this system of combined theory-experiment-simulation.
4. RESULTS AND DISCUSSION
Here, we present some examples of computational science using astronomical data and accelerator data. Regarding astronomical data, some areas of space are dense, and others are not, because space is uneven. As the universe is still being created, doubts have risen as to whether the mass of standard model matter is sufficient for creating the galaxy. Recent simulation results show that dark matter plays a central role in creating the universe. Two massive cosmological simulations have been performed by a group of Korean scientists who studied the clustering of galaxies on cosmic time and length scales (Kim et al. 2012), using 8,240 CPU cores and 8.7 and 15 TB of memory, respectively, to simulate the gravitational evolutions of 216 and 374 billion particles, respectively. To compare with future galaxy surveys, mock luminous red galaxies (LRGs) in the pace light cone space were constructed (Kim et al. 2012) using the large-scale computational science method described earlier. In the simulation results assuming that dark matter exists, the density of matter evolved substantially with time and showed very similar density between the creation of the universe and today’s large-scale galaxy structure (Cho 2016b).
The e+e- collider experiment is one example of computational science carried out using accelerator data. This aimed to discover new physical phenomena unexplained by the Standard Model. It tested related theories by comparing Monte Carlo simulation results based on phenomenological theories of the presence of dark matter and the corresponding accelerator data. Fig. 6 shows the dark matter production mechanism in the e+e- collider experiment (e.g., Belle II, International Linear Collider). It struggled to clarify the phenomenon of dark matter. Theories predicting unclarified regions were tested using simulation toolkits and Monte Carlo simulations were run using the KISTI supercomputer. The results of simulation outputs were then compared and analyzed to verify theories of dark matter and determine the characteristics of dark matter in search of new physical phenomena. Fig. 7 shows the Feynman diagrams of the Standard Model background of a dark mater event in the e+e- collider experiment. The channel is e+e- → ννγ. We study the decay of neutrinos as the main background event of dark matter decay.
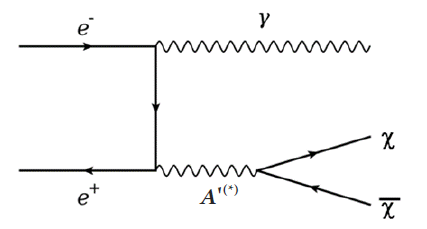
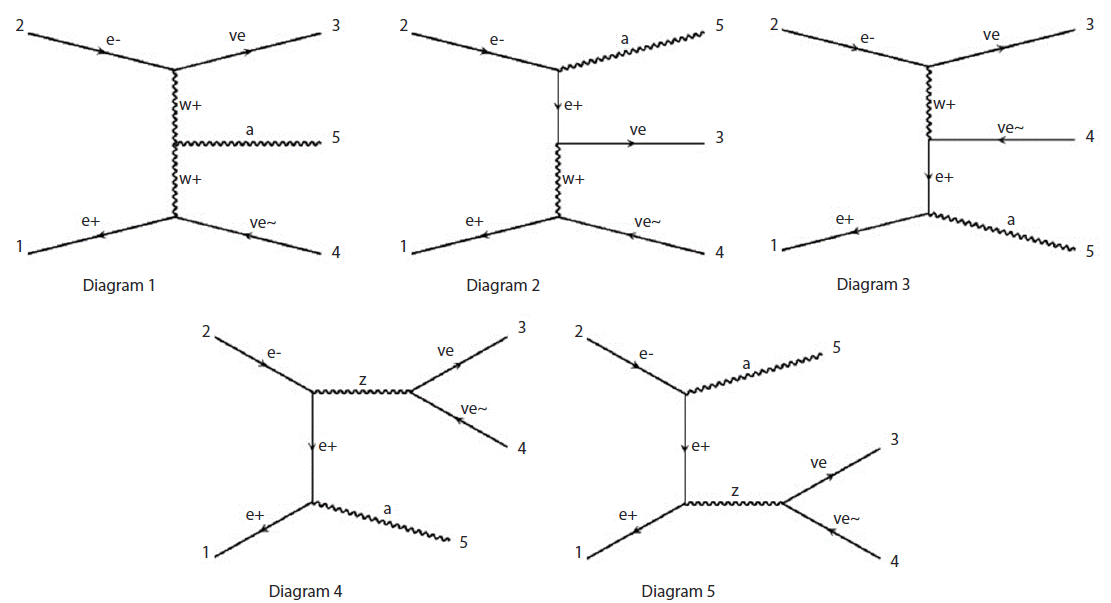
Fig. 8 shows the cross-section of the standard model background for e+e- → ννγ simulated by MadGraph (Alwall et al. 2014). The computational science results using astronomical data and accelerator data can be used as inputs for the dark matter research platform as constraint conditions.
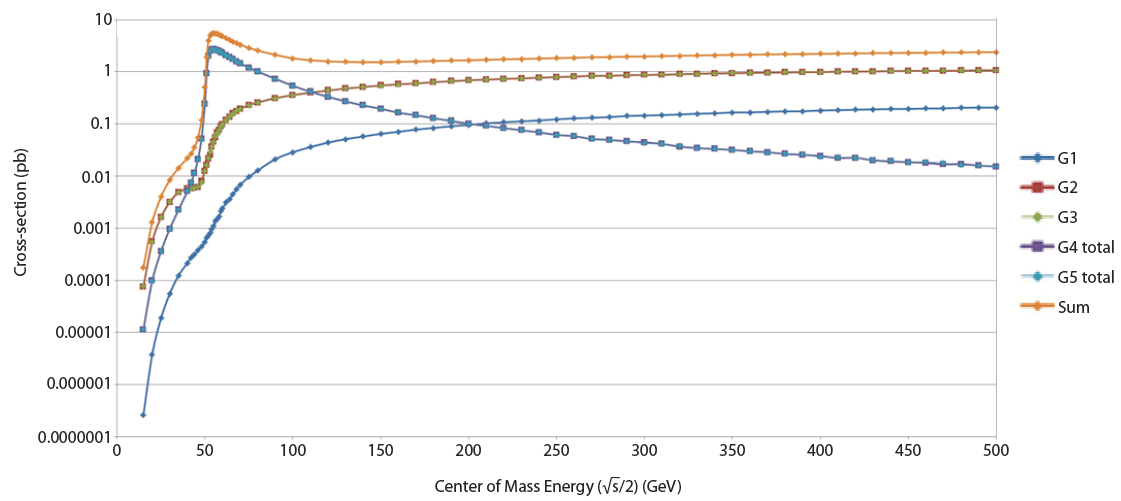
5. CONCLUSION
Computational science converging experiment–theory– simulation is required for research into dark matter detection, and relevant case studies are described in this paper. Computational science is essential for solving complicated problems in all fields beyond traditional science, including dark matter detection and core research on the evolution of the universe. Research on dark matter detection requires large data and computing resources. Moreover, software development required by the physics of dark matter detection are necessary. Since this cannot be performed by one particular research method, we suggest a dark matter research platform for synergizing all data from participating research institutes. Through it, we may prove the phenomenon of dark matter more efficiently by combining theoretical data, astronomical data, and accelerator data through computational science.